Well, it’s been one heck of a year. ::shaking head:: Although I love getting those end-of-year postcards from folks, I’ve never managed to make them. Instead of recounting my familial adventures and emotional trials and tribulations, I thought I could at least step back and reflect on some professional endeavors over the last year, many of which I did a lousy job of sharing when they happened.
1. I wrote three papers this year that I’m quite proud of.
“Statistical Imaginaries, State Legitimacy: Grappling with the Arrangements Underpinning Quantification in the US Census” is an analysis of four technical changes that the Census Bureau made / attempted to make in the last few decades: imputation, adjustment, swapping, differential privacy. Jayshree Sarathy and I examine the controversies around them with an eye towards why their complexity and visibility mattered. This is an extension of our earlier work on differential perspectives.
“The Resource Bind: System Failure and Legitimacy Threats in Sociotechnical Organizations” explores how time and money are weaponized by different actors involved in the Census Bureau and NASA in ways that threaten both the scientific work as well as the legitimacy of the organization. This was a Covid collaboration as Janet Vertesi and I spent long hours comparing our two different field sites to understand the constraints that each agency faced.
“Techno-Legal Solutionism: Regulating Children’s Online Safety in the United States” emerged when Maria Angel pushed me to step back from my fury over the motivations behind proposed laws like Kids Online Safety and Privacy Act in order to interrogate the proposed interventions. What we came to realize is that legal policy is now demanding technosolutionism, pushing tech companies to solve problems that they have no power over and no right to decide.
While these papers might not be as sexy as hot takes on AI, they all gave me great joy to write, both because my collaborators were awesome and because they involved deeper thinking about sociotechnical configurations. If you haven’t looked at them, please check them out! (And ping me if you can’t access them – I’m happy to send you a copy!)
2. I talked a lot. And in a lot of different contexts.
Taylor Lorenz and I explored the panic over social media and mental health together.
I appeared in the “Truth or Consequences” episode of “What’s Next? The Future with Bill Gates” (which you can find on Netflix).
I keynoted AI, Ethics, and Society where I built on the “abstraction traps” work I did with colleagues to highlight how the same traps and more are appearing in AI conversations. I repeated that argument a few more times in academic venues and now I need to write it all up.
I sat down with Tressie McMillan Cottom and Janet Vertesi at the Knight Foundation’s Informed conference where we explored what different theoretical insights have to offer practitioners.
I got to hang out with Kevin Driscoll at the Computer History Museum and discuss old skool online communities. (They called it The Prehistory of Social Media but that always makes me think of dinosaurs.)
DJ Patil and I spent an hour exploring what data scientists should know for his LinkedIn course on data science.
I gave the Wendy Michener Memorial Lecture at York University on how to be intentional about nurturing the social fabric that holds you. I also gave the Information Law and Policy Annual Lecture at the University of London on the importance of focusing on interventions, not solutions. (I hope to write these up shortly.)
I also bounced in other places around talking about such an eclectic set of topics that I’m starting to wonder who I am. I dove into synthetic data in Sweden, AI policy in Berlin, responsible AI in Edinburgh and Boston, survey methodology in Ithaca, political polarization in Cambridge, public trust in federal statistics in DC, online safety in a virtual conference, the politics of ignorance in Philadelphia, and youth mental health in multiple online venues. It’s been a weird year.
3. I failed to learn that policymakers don’t give a flying f&#$ about helping people.
Every time I get the pleasure of advising Crisis Text Line’s amazing team, I’m reminded of how much this network cares deeply about kids’ mental health and strategically leverages data to improve their services to meaningful help people.
And then I end up in policymaking conversations that are purportedly about helping people only to learn that no one wants to ground their interventions in evidence and, besides, helping people is only the rouse for other things. At peak frustration, I ranted a bunch of times. Two examples: 1) KOSA isn’t designed to help kids and 2) the ludicrous frame of harm that’s used in policy debates.
But let’s be honest, I mostly found myself screaming into the void to no effect.
Amidst this, I witnessed so many friends and collaborators be tortured by politicians, political operatives, and their various bulldogs. These acts of harassment were designed to silence my friends in the name of free speech. And it’s been devastating to see the effects.
It’s really hard not to get disillusioned. And still, I can’t resist trying to find a way to make a difference. Here’s hoping that I can find a new approach to my Sisyphean activities.
At least on the plus side, I’m actually enjoying the various conversations unfolding on Bluesky these days. It’s been nice to be back in online community with a range of people after running away from the high pitched hellzone that other sites had turned into.
4. I announced that I’m joining the Cornell faculty starting in July 2025.
This is a huge professional transition (and it means moving to Ithaca!) but I’m genuinely stoked about the new adventure, albeit sad to leave my MSR colleagues after 16 years. Still, ::bounce:: I can’t wait to see what this will lead to!
5. I read. A lot. And forced others to read too.
At night, my kids and I curl into a cuddle pile and read together. We read (and play board games) a lot. My favorite fun book this year was Trust by Hernan Diaz, which initially annoyed the heck out of me and then blew me away. On the professional side, I can’t stop thinking about how people throw chickens into airplane engines to test them. Thanks John Downer’s Rational Accidents. But there were also so many other good books. (I still use Goodreads to keep track.)
Since I hate reading alone, I’ve dragged so many people around me into book clubs all year long. I just want to publicly apologize to you all for the never-ending requests to read with me. But also, thanks!
6. Resilience is my word for 2025
In a meeting this week, Nancy Baym talked about how she wasn’t really into New Year’s resolutions. Instead, she chooses a word that she uses as her mantra for the year. A word that will work at multiple levels and invite deep reflection. I love this idea.
I’ve chosen the word “resilience” for next year. I’d like to think about how to ensure that I am personally resilient to the challenges and pressures that come with change and uncertainty. I’d also like to think about how to support the development of resilience in people and organizations around me.
So I will leave you with this thought: what’s your word for 2025?
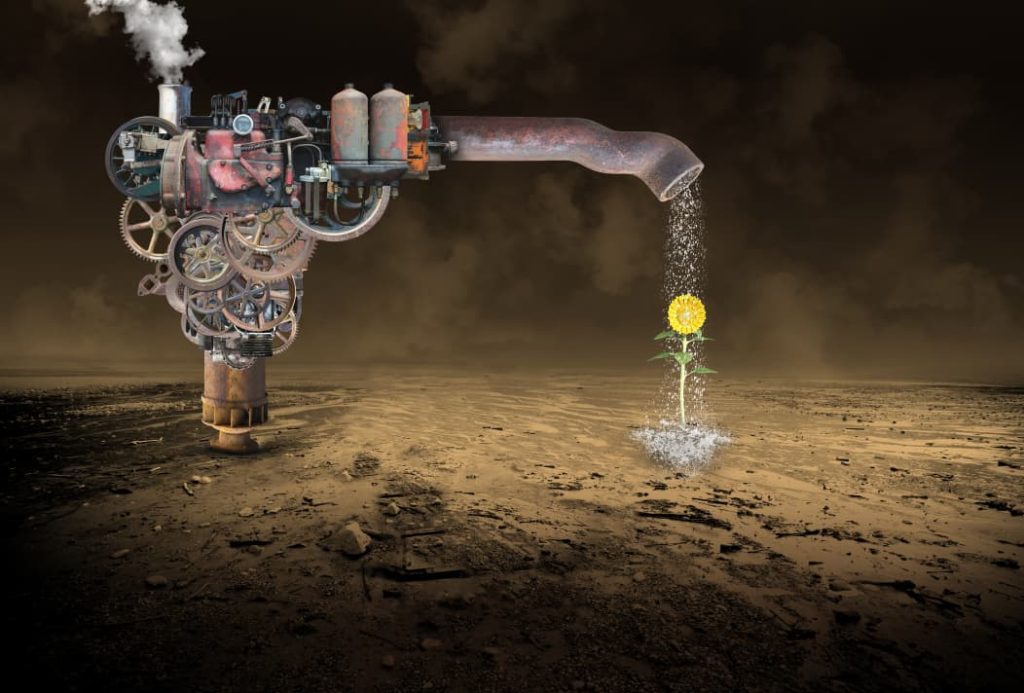